Machine learning to address environmental problems
Keywords: Machine learning, deep learning, remote sensing, archaeology, environment, GIS, spatial, climate change


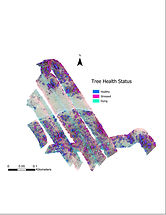
Postdoctoral Work:
​
I apply deep learning to sidescan sonar and magnetometer data for aircraft detection. As part of an interdisciplinary team with expertise in remotely sensed data collection, unmanned underwater vehicle (UUV) operation, oceanography, archival research, and technical scientific diving, we are focused on finding underwater aircraft wrecks around the world that may contain the remains of missing-in-action US service members.
​
Other Projects:
​
I am working on a project applying an updated version of the model used for my shipwrecks and Maya dissertation work to to detection of ancient Romanian burial mounds in airborne natural color imagery using vegetation indices. We are currently in the process of acquiring high-resolution spectral satellite imagery for the entire country, which would enable us to create a comprehensive inventory of Romanian burial mounds. As part of this, we will develop a custom vegetation index for use with the spectral imagery and compare the performance of the spectral-derived index to that of the natural color-derived index. Some of the data for this project were labeled by a high school history class during their archaeology unit. We used QGIS to label ancient Romanian burial mounds in airborne imagery for input into the machine learning model. The students had no GIS or remote sensing background, so the class introduced them to new fields while also providing me with a fully labeled training dataset.
​
I am also interested in broader, non-archaeological, applications of machine learning to address environmental problems related to climate change. This includes a project focused on using machine learning and hyperspectral imagery to assess tree health. I am also just beginning a project focused on the use of machine learning to exploit years of video data collected by penguin-mounted video cameras to inform analyses of the effect of a changing Antarctic climate on penguin foraging habits.
​
​
​
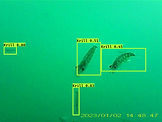_krill.jpg)
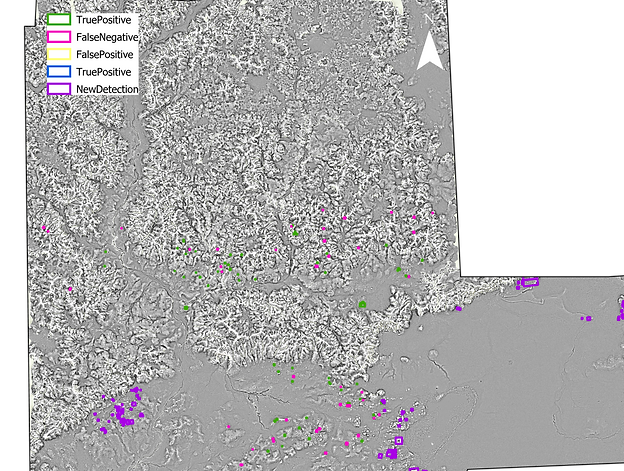
Phd dissertation research: machine learning for archaeological feature detection
Archaeological features can be impossible to map comprehensively and systematically across the landscape because the process is time-consuming, labor-intensive, and features are often obscured or difficult to access. Lidar and sonar imagery, which can penetrate dense tropical forest canopy and waterbodies, respectively, combined with powerful new machine learning models, enable a new approach to site survey. The work presented in this dissertation focuses on strengthening this approach by creating accurate models that are both generalizable and transferable, as well as defining the role of machine learning in archaeology. First, we introduce a deep learning framework to accurately detect submerged shipwrecks around the coast of the United States. We then use the same model framework, along with an expanded and improved imagery preprocessing and relief visualization methodology, to detect ancient Maya structures across three densely vegetated archaeological sites in Guatemala and Mexico. We conclude with a random forest model that uses a very small amount of training data to detect areas that may contain potential cave entrances at a Maya archaeological site in Belize. This work shows that machine learning, and in particular deep learning, is capable of systematically mapping diverse features across a heterogenous landscape. When features are more morphologically uniform, such as with cave entrances, and when the training data quantity is small, simpler modeling approaches, such as random forest, may be appropriate or necessary. This work also demonstrates the generalizability of a broadscale machine learning model across the Central American landscape for Maya structure detection, as well as the transferability of the same model framework from the shipwreck detection project to the Maya structure detection project. It is important to note, however, that the role of machine learning in archaeology is not to replace more well-established methods of site survey, but rather to complement them. Machine learning offers the strongest contribution to archaeological survey when implemented in conjunction with fieldwork and manual imagery-based survey. We believe this threefold approach is the future of archaeological site survey.